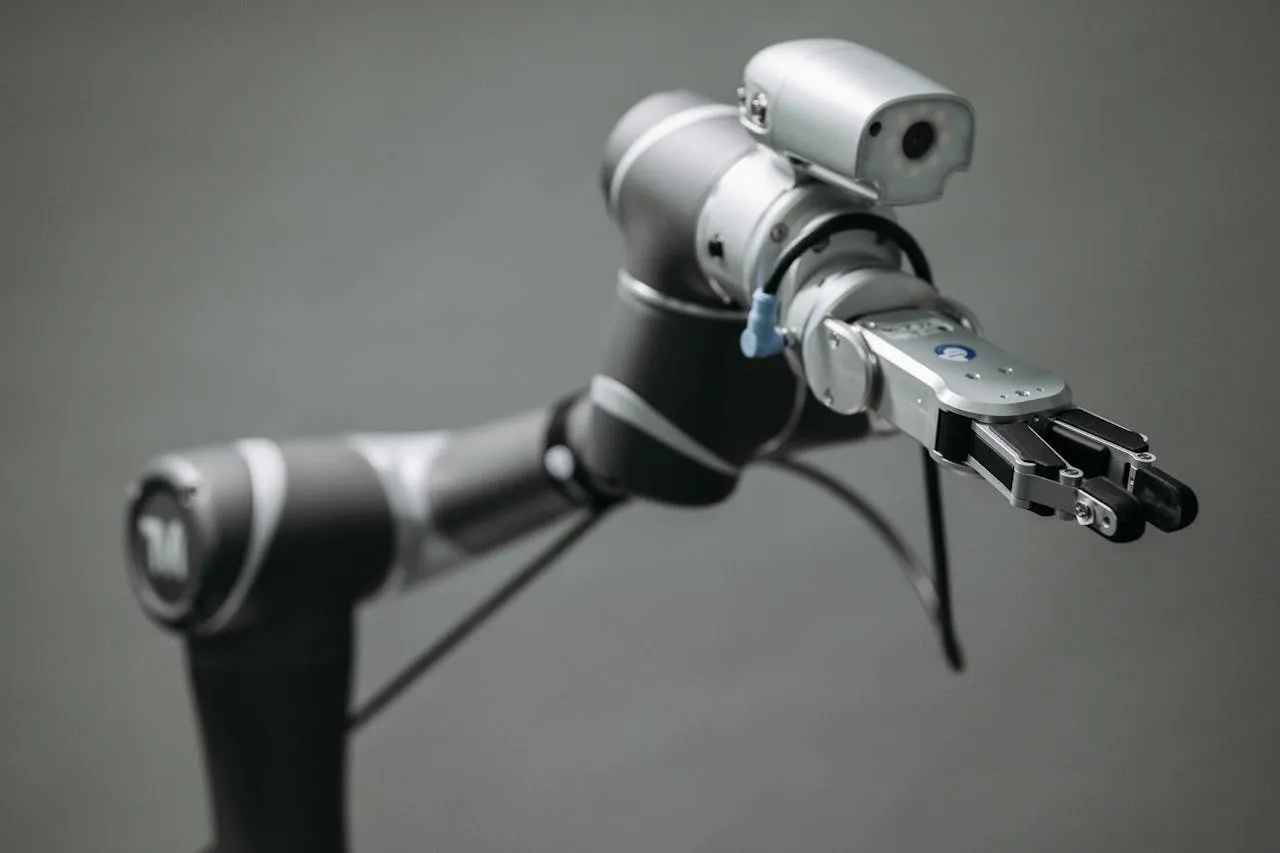
NTT Research and Harvard Scientists Leverage Machine Learning to Advance Biohybrid Ray Development
NTT Research, Inc., a division of NTT (TYO:9432), and the Harvard John A. Paulson School of Engineering and Applied Sciences (SEAS) have made significant advancements in biohybrid robotics by applying machine-learning directed optimization (ML-DO). Their latest research, published in Science Robotics under the title “Bioinspired Design of a Tissue Engineered Ray with Machine Learning,” showcases how this approach efficiently identifies high-performance design configurations for biohybrid robots. The study demonstrates that ML-DO can significantly improve the swimming efficiency of biohybrid rays compared to conventional biomimetic methods.
The research team, led by Harvard SEAS Postdoctoral Fellow Dr. John Zimmerman, included NTT Research Medical and Health Informatics Scientist Ryoma Ishii, Harvard SEAS Tarr Family Professor of Bioengineering and Applied Physics Kevin Kit Parker, and members of the Harvard SEAS Disease Biophysics Group led by Parker. Their findings indicate that ML-DO enables a more effective and computationally efficient way of designing biohybrid robotic structures by optimizing muscular function and morphology.
Overcoming Limitations of Biomimetic Design
Traditional biomimetic design focuses on replicating biological structures to create functional devices. While this method has yielded various biohybrid robots, it presents notable limitations. For biohybrid organisms that resemble batoid fishes, such as skates and rays, a wide range of natural aspect ratios and fin morphologies exist. However, mimicking these structures does not necessarily translate into optimal performance.
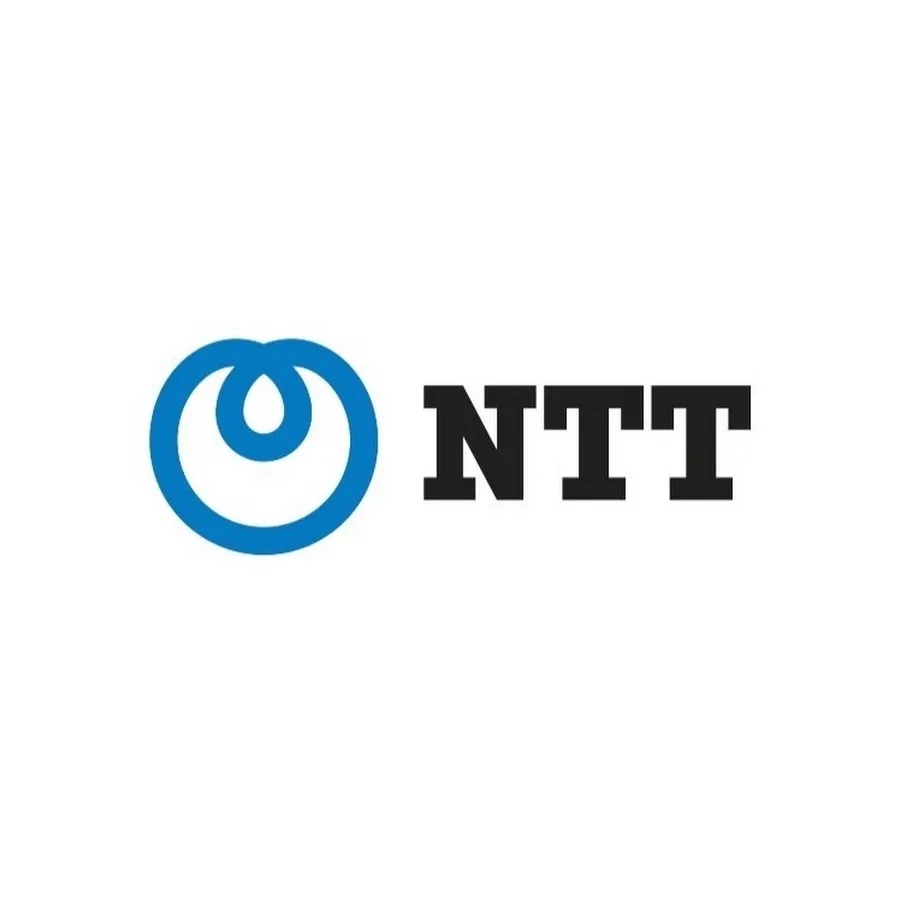
One of the primary challenges of biomimetic design is that it often overlooks biomechanical and hydrodynamic constraints. These natural forces determine how efficiently an organism can swim based on its size, muscle mass, and fin movements. As a result, biomimetic biohybrid rays tend to exhibit inefficient swimming mechanics, limiting their speed and agility. This inefficiency underscores the need for a more advanced design approach, such as ML-DO, to better optimize robotic structures for performance.
Machine Learning Unlocks Design Breakthroughs
The multidisciplinary nature of biohybrid robot development necessitates computationally intensive modeling. However, the researchers hypothesized that ML-DO could streamline the design process by rapidly identifying optimal fin geometries that maximize swimming efficiency. Their hypothesis was partially based on trial functions demonstrating that ML-DO outperformed other leading methods by approximately 40% in recognizing high-rank sequences.
To test their hypothesis, the research team followed a three-step approach:
- Algorithm Development: They designed an algorithm capable of representing a wide variety of fin geometries.
- Search Optimization: They formulated a generalized ML-DO strategy to efficiently navigate the complex, discontinuous configuration space of possible fin designs.
- Performance Validation: They applied their methodology to identify biohybrid fin structures that promoted smooth, efficient swimming.
Their findings revealed that fins with large aspect ratios and finely tapered tips consistently outperformed other designs. These optimized fins preserved their functionality across multiple swimming scales and significantly improved efficiency. NTT The researchers then built biohybrid mini-rays using engineered cardiac muscle tissue, demonstrating that these new designs doubled swimming efficiency compared to previous biomimetic models.
The Road Ahead: Further Refinements and Applications
While the ML-DO-driven biohybrid rays outperformed previous biomimetic models, they still exhibited slightly lower efficiency than their natural marine counterparts. To fully match the efficiency of biological organisms, additional refinements are needed. The researchers plan to further investigate how machine learning can fine-tune muscle dynamics and hydrodynamic interactions in biohybrid structures.
Beyond robotic swimming efficiency, this research has far-reaching implications. The development of biohybrid robotics could revolutionize multiple fields, including:
- Environmental Monitoring: Biohybrid robots could serve as remote sensors for oceanic and aquatic environments, collecting data in ways that traditional robotic systems cannot.
- Exploration and Surveillance: These robots could be deployed in hazardous or inaccessible environments, such as deep-sea trenches or contaminated water bodies.
- Medical and Therapeutic Applications: The principles behind ML-DO-driven biohybrid robots could inform the development of biohybrid medical devices, such as soft robotic implants or therapeutic delivery vehicles.
Additionally, ML-DO offers a new paradigm for studying evolution and biomechanics. By simulating selective pressures akin to natural evolution, researchers can better understand how biological tissues and organisms develop optimal structures and functions. This knowledge could have profound implications for fields such as developmental biology, organ biofabrication, and tissue engineering.
Strengthening Research Collaboration
The success of this project highlights the growing synergy between NTT Research and Harvard SEAS. Their collaboration, established through a three-year joint research agreement in 2022, aims to deepen our understanding of NTT cardiac physiology and biohybrid devices. This initiative includes engineering a model of the human heart, studying fundamental laws governing muscular pumps, and applying findings to develop a cardiovascular bio-digital twin model.
Professor Kevin Kit Parker emphasized the importance of their partnership, stating, “The Harvard Biophysics Group entered into a joint research agreement with NTT Research two years ago aiming to fundamentally interrogate our understanding of cardiac physiology, with an eye towards advancing the development of biohybrid devices, including biohybrid robotics and biohybrid human heart. This paper indicates the positive progress our shared research has achieved so far, and I am excited to see what the future of our collaboration has in store.”
The latest research by NTT Research and Harvard SEAS underscores the power of machine learning in optimizing biohybrid robot design. By addressing the limitations of conventional biomimetic approaches, ML-DO offers a more efficient and scalable way to engineer high-performance biohybrid systems. While further refinements are necessary to match natural efficiency, the progress made thus far opens new possibilities for biohybrid robotics in environmental, medical, and industrial applications.
As this field continues to evolve, the integration of machine learning with bioengineering promises to unlock new frontiers in robotics, medicine, and beyond. The continued collaboration between academic institutions and industry leaders like NTT Research will likely accelerate these advancements, bringing biohybrid technology closer to real-world applications.